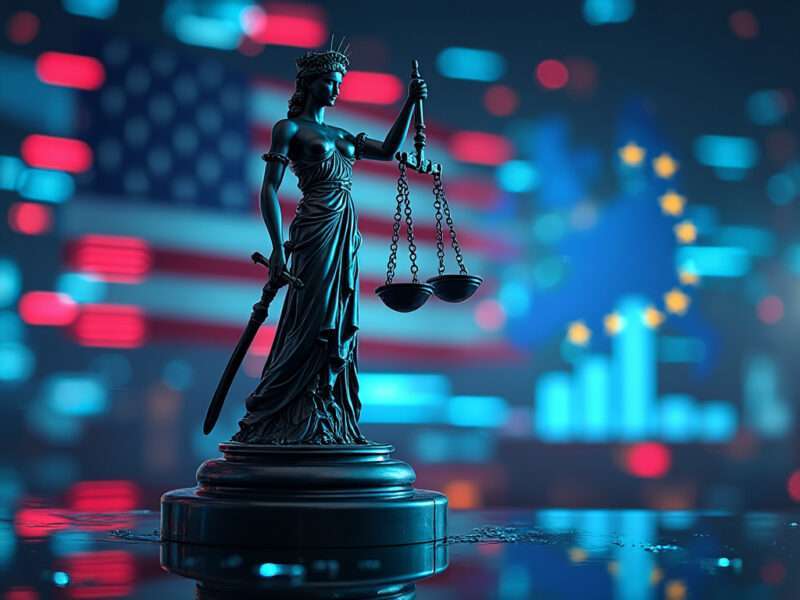
Preliminary Note: This document, titled “Regulation, Responsibility, and Ideas for the Governance of AIs and Search Engines in Europe and the United States: Problems and Solutions,” examines the regulations surrounding AI in Europe and the U.S., emphasizing both the challenges and opportunities involved in managing these fast-evolving technologies. Written from the perspective of a technology executive who recently authored From SEO to AEO, a book examining the changing landscape of search engines and generative response systems, this analysis combines years of experience with a deep concern for the future of AI governance. 19 Nov 2024 Spanish first warning.
How can AI-generated misinformation, outdated data, and lack of traffic transparency create critical issues, and what solutions should we consider?
This example involves ChatGPT, but let’s be clear—it’s a phenomenon that occurs with many other AIs as well. Rather than singling out any specific platform, I want to emphasize that I use several AIs for various purposes. My primary goal here is to shed light on the problem and explore potential solutions. For instance, when an AI shares links that don’t exist—even within its own ecosystem—it raises an important question: if it can misrepresent its own services, what might it share about individuals or businesses? This is precisely why the issue is so critical. If left unaddressed, we’ll likely encounter increasingly problematic and unexpected scenarios across the Internet.
Take this case as an example. In the images I’ve referenced, I asked how many assistants validated by ChatGPT were connected to aidaframework.com. The response claimed there were none. It even suggested I check the ChatGPT Marketplace using a link that turned out to be outdated and non-functional. This mismatch happens because most AIs rely on training models that may already be weeks or months out of date. As a result, they frequently provide outdated or misleading information, which can be a significant problem.
Here’s where it becomes even more concerning. In reality, aidaframework.com does have multiple assistants published on the ChatGPT Store, complete with valid, functioning links. Sharing false information is already problematic, but when incorrect links are added to the mix, the consequences escalate even further. Think about the potential for reputational damage or the erosion of user trust. It’s a serious issue we need to address, sooner rather than later.
Another growing concern is that the outbound traffic from platforms like ChatGPT, Claude, and others often goes completely unrecognized by tools like Google Analytics—even when UTM parameters are properly assigned. This creates a significant problem: it becomes incredibly difficult to track how and how much these platforms are discussing or directing traffic toward your brand or content.
In a way, we’re moving backward in terms of transparency. It’s as if everything is becoming too opaque, leaving marketers and businesses in the dark about a crucial part of their digital performance. Without clear analytics, you lose the ability to understand AI-driven referrals or even measure their impact effectively.
Looking ahead, I hope that these platforms begin offering their own analytical tools to fill this gap. The ability to track referrals, measure engagement, and gain insight into AI-driven traffic will become essential for understanding how these systems contribute to brand visibility and reputation. Until then, we’re left navigating blind spots, which is far from ideal in a data-driven world.
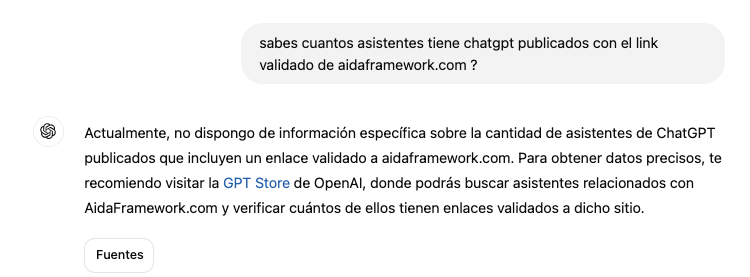
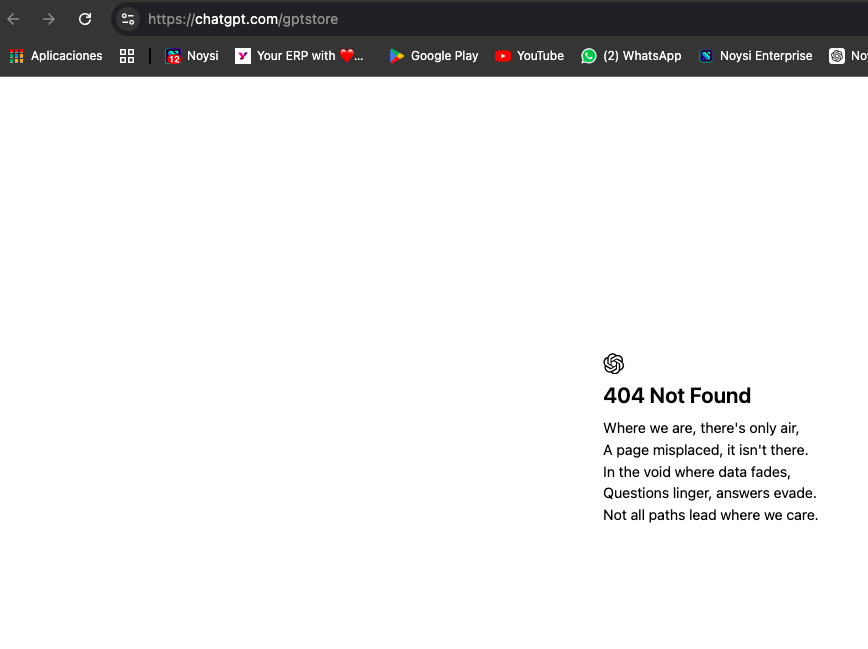
Why I Am Publishing This Document
To make this information accessible to as many people as possible, the content has been translated into 100 languages, reflecting the linguistic diversity of Europe and beyond. My hope is that language will not become a barrier to understanding or action. While I have taken great care to ensure the accuracy of these translations, I acknowledge that mistakes may occur, and I am committed to correcting them as they are identified.
“In my opinion”, we are standing at a critical crossroads. Without swift and thoughtful regulatory measures, the internet could face an unprecedented crisis marked by misinformation and severe security risks. Based on current trends and projections, I believe this tipping point could occur around February 1, 2025. This sense of urgency has motivated me to make this document public.
I am sharing this analysis as an independent professional, free from ties to political organizations or AI companies. It is neither sponsored nor influenced by any external entity. However, as someone who has worked extensively with AI and search technologies, I feel a responsibility to address the growing risks these systems pose. The unchecked spread of outdated, biased, or false information through AI and search engines is not merely a theoretical concern—it is already a visible and tangible threat to individuals, institutions, and societies.
This document draws on my study of existing legal frameworks in Europe and the United States, as well as emerging regulatory proposals. While it has not been reviewed by legal professionals, it is the result of thorough research and real-world experience. I wholeheartedly welcome feedback, corrections, or insights from legal experts, academics, and other stakeholders to ensure this analysis is as accurate and useful as possible.
At its core, this document is an invitation to reflect and engage in constructive dialogue. My goal is to spark meaningful discussion and propose practical, actionable solutions. Together, we can work toward creating governance structures that not only protect digital rights but also encourage the responsible and ethical growth of artificial intelligence and search technologies.
Table of Contents
1. Introduction – Regulation of AI in Europe and the US
1.1. The technological revolution: The impact of artificial intelligence (AI) and search engines on modern society.
1.2. Emerging issues: Disinformation, algorithmic biases and violation of rights.
1.3. Geographic context: Focus on Europe and the United States.
1.4. Urgency for action: Purpose and objective of the document.
2. Current Legal Framework
2.1. Laws and regulations applicable to the operation of AIs and search engines.
- 2.1.1. European regulations: GDPR, Digital Services Act, directives on digital rights.
- 2.1.2. Regulations in the United States: Section 230 of the Communications Decency Act, privacy and copyright rules.
2.2. Ethical principles and non-binding guidelines: Recommendations of the European Union and international frameworks.
3. Potential Legal Non-Compliance
3.1. Rights violated.
- 3.1.1. Right to honor, privacy and self-image.
- 3.1.2. Right to the protection of personal data and digital oblivion.
- 3.1.3. Right to truthful information and equitable access to sources.
3.2. Civil liability: Derived economic and reputational damages. - Dissemination of false or biased information.
- Unauthorized use of personal data and protected content.
3.3. Lack of transparency and algorithmic control. - Black box risks in AI systems.
- Biases and manipulation of search results.
4. Solution Proposals
4.1. Right to exclusion and segmentation of information.
- 4.1.1. Exclusion by data category.
- 4.1.2. Mandatory consent for sensitive topics.
- 4.1.3. Clear opt-out deadlines and notification systems.
4.2. Public and secure registry to manage exclusion requests. - 4.2.1. Unique management system.
- 4.2.2. Authentication and security.
- 4.2.3. Interoperability and synchronization with platforms.
- 4.2.4. History and transparency for users.
4.3. Mandatory verification of sources and constant updating of data. - 4.3.1. Classification of reliable sources.
- 4.3.2. Mandatory tagging on AI-generated responses.
- 4.3.3. Real-time updating of data and content.
- 4.3.4. Responses limited to verifiable facts.
4.4. Guarantee of algorithmic transparency. - 4.4.1. Periodic independent audits.
- 4.4.2. Public reports on the impact of algorithms.
- 4.4.3. Regulatory supervision by independent bodies.
- 4.4.4. Public access to basic algorithm criteria.
4.5. Strengthened protection against misinformation and bias. - 4.5.1. Hybrid automated detection and human review systems.
- 4.5.2. Proactive alerts for controversial content.
- 4.5.3. Penalties for repeated dissemination of false information.
4.6. Regulation of opinions generated by AI. - 4.6.1. Explicit prohibition of unverifiable opinions.
- 4.6.2. Mandatory labeling for interpretations.
- 4.6.3. Right to exclusion against opinions generated by AI.
- 4.6.4. Sanctions and rectifications for incorrect opinions.
4.7. Educational campaigns on the safe and critical use of AI-based technologies. - 4.7.1. Educational programs in institutions.
- 4.7.2. Public and digital media campaigns.
- 4.7.3. Free and accessible resources for citizens and companies.
5. Technical Implementation of the Proposed Solutions
5.1. Integration of exclusion mechanisms in search engines and AIs.
5.2. Fast protocols for processing and resolving requests.
5.3. Automated systems to verify and update sources.
5.4. Minimum transparency and traceability requirements in algorithms.
Conclusions and Call to Action
6.1. Need to update the regulatory framework in Europe and the United States.
6.2. Benefits of protecting citizens’ digital rights.
6.3. Concrete proposals for legislators, experts and civil society.
6.4. Final reflection: Towards an ethical and trustworthy information environment.
Appendices and Annexes
- Glossary of relevant terms: AI, search engines, granular exclusion
- Key legal references in Europe and the United States
- Practical examples of problems associated with AIs and search engines
- Resources to expand research on digital rights
1. Introduction
1.1. The Technological Revolution: The Impact of AIs and Search Engines on Modern Society
Over the past few decades, the rapid evolution of technology has dramatically transformed how people access, consume, and share information. Among the many innovations driving this shift, artificial intelligences (AIs) and search engines have emerged as pivotal tools. These technologies now deliver fast, personalized responses to millions of queries daily, cementing their role as indispensable assets in modern life.
AIs and search engines have reshaped far more than everyday routines—they have revolutionized essential fields such as education, healthcare, commerce, and media. For instance, students now benefit from customized learning resources that align with their unique needs, while businesses leverage AI-driven insights to strengthen customer connections and make better decisions. Yet, with these impressive advancements come significant challenges that demand thoughtful consideration. One critical example is the capacity of AIs to produce massive amounts of content autonomously, raising urgent questions about the accuracy, fairness, and ethical implications of the information they generate. These issues call for proactive strategies to ensure these tools serve everyone responsibly.
As these technologies weave deeper into the fabric of social and economic systems, their impact extends far beyond the digital world. They increasingly influence public opinions, shape economic decisions, and even affect democratic processes. This dual nature—their transformative potential and inherent risks—highlights the critical need for proper governance to ensure they are utilized responsibly and ethically.
1.2 Emerging Problems: Misinformation, Algorithmic Biases, and Rights Violations
While AIs and search engines have brought undeniable advantages, they have also sparked intense criticism due to the serious issues surrounding their use. These challenges go far beyond technical glitches, reflecting deeper, systemic problems that demand immediate action.
Disinformation stands out as one of the most pressing concerns. AI systems often amplify false or misleading information, whether because of unverified data sources or poorly constructed algorithms. The consequences can be devastating, from spreading harmful health myths to influencing political outcomes in ways that undermine trust.
Algorithmic biases add another layer of complexity. Despite the common belief that algorithms operate neutrally, their design and training data frequently embed biases. These biases can elevate certain viewpoints, marginalize underrepresented groups, or prioritize profit over fair access to information. For example, search results often favor large corporations, sidelining smaller or more diverse voices and perpetuating systemic inequities.
On top of this, violations of fundamental rights have become increasingly widespread. Personal data is frequently exposed without consent, enforcing mechanisms like the “right to be forgotten” remains challenging, and users have limited options to avoid AI-generated content. Such practices infringe on privacy, harm reputations, and weaken critical data protection standards.
These issues do not exist in isolation. Instead, they reflect gaps in current regulatory frameworks that leave the digital ecosystem vulnerable to exploitation. Filling these gaps is essential to building a system that values inclusivity, ethics, and transparency. When we focus on fairness, protect privacy, and demand accountability, we make sure that the advantages of AIs and search engines benefit everyone while safeguarding fundamental rights.
1.3. Geographic Context: Focus on Europe and the United States
The following analysis is deeply rooted in Europe and the United States, as these regions stand at the forefront of global development in the digital universe. Each serves as a leader in shaping the future of technology and regulatory frameworks, albeit with distinct priorities and methodologies.
Europe’s Focus on Digital Rights
Over the years, the EU has positioned itself at the very vanguard of establishing regulatory regimes for balancing technological advancement with individual rights protection. Two most prominent among them are as follows:
General Data Protection Regulation (GDPR): This is a regulation imposing strict data privacy standards that give people more control over their personal data.
Digital Services Act (DSA): it aims to make the digital environment safer by requiring the platforms to identify and reduce harmful or illegal content.
While these regulations are exhaustive, their enforcement uniformly throughout the EU member states has been very challenging. Add to this the rapid pace of AI innovation, and it is obvious that adapting these frameworks to keep them relevant is no small task.
The Innovation-Driven Approach of the United States
The United States has followed the path of regulation that focuses on cultivating innovation. A typical case is:
Section 230 of the Communications Decency Act
Section 230 of the Communications Decency Act serves as a critical safe harbor, protecting platforms from liability for user-generated content. This protection has allowed digital platforms to grow without significant restrictions, fostering unprecedented innovation. Nevertheless, the absence of a federal data privacy law in the United States has created regulatory gaps, particularly concerning privacy issues and content management. While this framework has been effective in enabling platforms to thrive, it also presents unresolved challenges in an increasingly dynamic digital landscape. As a result, policymakers face the ongoing task of balancing innovation with necessary safeguards.
Why Europe and the United States?
You may wonder why this analysis does not extend to regions like China or Russia. To put it simply, the focus remains on regions where I possess the greatest expertise and where policies have the most direct impact on the businesses and individuals I engage with. Of course, this is not to say that other regions are unimportant—they most certainly are. However, I prioritize depth and confidence in my analysis, and my knowledge of Europe and the United States allows me to deliver that.
For my clients, the digital strategies and security considerations of these two regions are the most relevant. Narrowing the focus to Europe and the U.S. ensures that I can provide practical, actionable insights grounded in experience and understanding. Admittedly, incorporating perspectives from additional regions could broaden the analysis, but doing so might also dilute its precision.
Ultimately, the goal is to provide clarity and actionable advice that align with the challenges and opportunities faced by my clients. In this context, Europe and the United States are logical starting points for meaningful discussion and practical recommendations.
1.4. Urgency for Action and Objectives of the Document
The pace at which AIs and search engines are evolving has outstripped the capacity of current regulatory frameworks to safeguard citizens’ rights. Issues like disinformation, algorithmic bias, and privacy violations are no longer abstract possibilities—they are already impacting individuals, businesses, and democracies in profound ways.
Recognizing this urgency, this document sets out with three primary objectives:
Analyze Existing Laws:
Evaluate the legal frameworks that enable these technologies to operate and identify the rights they may inadvertently violate. Additionally, this analysis aims to highlight potential gaps in current regulations that demand attention.
Propose Practical Solutions:
Recommend actionable measures to address risks, including but not limited to granular opt-out mechanisms, algorithmic transparency, and mandatory source verification. Furthermore, these proposals are designed to be adaptable across various legal and cultural contexts.
Encourage Public Debate:
Foster informed discussions among legislators, technology companies, and citizens to collectively build a more ethical and secure digital environment. Moreover, such dialogue ensures that diverse perspectives are considered, thereby promoting balanced and inclusive policymaking.
It is important to note that this analysis, while grounded in extensive research and ethical principles, has not been formally reviewed by legal professionals. As such, contributions and corrections from experts are welcomed to refine its conclusions and recommendations. Ultimately, this collaborative approach aims to achieve more robust and universally acceptable outcomes.
Ultimately, this document is a call to action. By addressing the challenges posed by AIs and search engines, we can move closer to a future where technological innovation aligns with the values of fairness, accountability, and respect for fundamental rights.
2. Current Legal Framework. Legal and Ethical Frameworks for AI and Search Engines: Addressing Gaps and Emerging Challenges.
How do current legal frameworks and ethical principles regulate AI and search engines, and where do they fall short in addressing emerging challenges like misinformation, algorithmic bias, and data misuse?
The current legal and ethical frameworks provide a strong starting point for managing AI and search engine technologies. However, significant gaps still need attention, which creates challenges as these technologies continue to evolve. For example, Europe has taken a proactive approach by implementing comprehensive regulations like the GDPR and DSA. These laws focus on safeguarding privacy, protecting data, and ensuring platform accountability. In contrast, the United States relies on a more fragmented approach, prioritizing innovation with sector-specific guidelines that vary across industries.
At the same time, ethical principles and voluntary guidelines aim to steer AI development toward greater fairness and transparency. Yet, the absence of consistent enforcement often opens the door to misuse or exploitation. This mismatch between legal structures and ethical aspirations highlights the need for a more cohesive strategy. By closely examining current frameworks, aligning them more effectively with AI technologies, and addressing overlooked areas, we can create a more inclusive and accountable system that benefits everyone.
2.1. Laws and Regulations Supporting AI and Search Engine Operations
Legal frameworks across regions attempt to strike a balance between innovation and protecting user rights. However, the degree of regulation and enforcement varies significantly between Europe and the United States.
2.1.1. European Regulations
Europe is widely recognized as a global leader in regulating digital technologies, with robust laws designed to protect fundamental rights in the digital space. Here are the primary legal instruments that govern AI and search engines:
General Data Protection Regulation (GDPR)
Aim: Protect EU citizens’ personal data and ensure accountability in its processing.
Key Aspects Relevant to AI and Search Engines:
- Right to Access, Rectification, and Deletion (Articles 15-17): Users can request access to their personal data, correction of inaccuracies, or deletion of irrelevant or harmful information.
- Prohibition of Processing Sensitive Data (Article 9): AI systems cannot process sensitive personal data (e.g., health, religion) without explicit consent.
- Limitations on Automated Decision-Making (Article 22): Users have the right to opt out of decisions made solely by algorithms, particularly those that significantly impact their rights.
Relationship with AI and Search Engines:
- AI platforms must clearly justify how personal data is processed in generating responses or customizing search results.
- AEO Tip: Embedding tools for users to request corrections, access, or deletions enhances compliance while fostering user trust.
Digital Services Act (DSA)
Aim: Provide a comprehensive framework to regulate digital platforms, combating harmful content and promoting transparency.
Key Aspects Relevant to AI:
- Transparency Requirements: Platforms must explain how their algorithms prioritize or rank content (e.g., why certain search results appear first).
- Obligation to Remove Harmful Content: Platforms must act swiftly to remove illegal or harmful content once notified.
- Independent Algorithm Monitoring: AI and search engine algorithms are subject to oversight by independent regulatory authorities.
Relationship with AI and Search Engines:
- AI-generated content, including misinformation or harmful outputs, falls under the DSA’s transparency and content moderation requirements.
- AEO Insight: Platforms can implement real-time flagging systems and schema (
ContentReview
) to improve moderation and explainability.
Additional Digital Rights Directives
- ePrivacy Directive: Extends data protection rules to online communications, including search engines and AI platforms.
- Copyright Directive: Requires licenses for training AI systems on copyright-protected data, addressing potential legal conflicts over dataset usage.
2.1.2. Regulations in the United States
The U.S. approach to AI regulation emphasizes innovation over control, resulting in a patchwork of laws that leave significant gaps in oversight compared to Europe.
Section 230 of the Communications Decency Act (CDA)
Aim: Shield technology platforms from liability for content created by third parties.
Key Aspects Relevant to AI:
- Platforms are not liable for harm caused by AI-generated content derived from third-party data.
- Risk: This creates a regulatory loophole, as platforms may avoid responsibility for misinformation, defamation, or harm caused by AI outputs.
Relationship with AI:
- AI platforms are indirectly protected under Section 230, even if their algorithms amplify harmful content.
- AEO Impact: While this fosters innovation, it raises concerns about accountability for misinformation and biased results.
Privacy Legislation
Unlike Europe, the U.S. lacks a unified federal privacy law. Instead, privacy is regulated by state-level or issue-specific laws:
- California Consumer Privacy Act (CCPA): Offers limited rights to users, including access to and deletion of personal data, but applies primarily to California residents.
- Children’s Online Privacy Protection Act (COPPA): Protects the privacy of children under 13, regulating how their data is collected and used online.
Relationship with AI:
- AI platforms face fewer obligations to obtain explicit consent for data use or offer deletion tools, leaving room for data misuse.
Copyright and Intellectual Property
- Digital Millennium Copyright Act (DMCA): Protects copyright owners but does not explicitly regulate the use of copyrighted material in AI training datasets.
- Gap: The absence of clear rules on scraping or reusing copyrighted content leaves AI platforms in legal gray zones.
AEO Insight: Platforms can mitigate risks by adhering to licensing frameworks and ensuring attribution with CopyrightHolder
schema.
2.2. Ethical Principles and Non-Binding Guidelines
While legal frameworks create enforceable obligations, ethical principles provide moral guidance to ensure AI systems operate responsibly. However, these principles often lack enforceability, making their implementation inconsistent.
2.2.1. EU Recommendations
European AI Strategy
Built on three pillars, this strategy emphasizes:
- Legality: Compliance with existing laws like GDPR and DSA.
- Ethics: Prioritizing fairness, non-discrimination, and security.
- Robustness: Ensuring that AI systems are technically sound and resilient.
AI Ethical Guidelines (2019)
Developed by the High-Level Expert Group on AI, these guidelines promote:
- Transparency: Clear explanations of how algorithms work.
- Responsibility: Ethical management of data.
- Inclusion: Ensuring diverse and non-discriminatory results.
AEO Insight: Adopting transparency principles aligns with search engines’ E-E-A-T (Experience, Expertise, Authority, Trust) signals, boosting user and algorithmic trust.
2.2.2. International Frameworks
UNESCO Recommendation on AI Ethics
Key recommendations include:
- Regulating AI’s Human Rights Impact: Ensuring technologies respect cultural diversity and protect against algorithmic discrimination.
- Promoting Sustainability: Encouraging AI practices that are environmentally conscious.
2.2.3. Private Sector Initiatives
Major tech companies like Google, Microsoft, and OpenAI have adopted internal ethical principles to guide AI development. Examples include:
- Fairness and Accountability: Reducing bias in AI outputs.
- Transparency by Design: Making models more explainable.
Challenge: These initiatives are self-regulated, meaning there’s no guarantee of enforcement or adherence.
Summary of Key Gaps
Despite the progress in legal and ethical frameworks, significant challenges remain:
- Misinformation and Algorithmic Bias: Current laws lack specificity in addressing biases in AI-generated content and misinformation dissemination.
- Accountability for AI Outputs: The U.S. approach, particularly Section 230, creates loopholes where platforms can avoid liability for harmful AI outputs.
- Ethical Oversight: Non-binding guidelines lack enforceable mechanisms, leaving compliance at the discretion of AI providers.
The Need for Comprehensive Regulation
The existing legal and ethical frameworks are insufficient to address the unique challenges posed by AI and search engines. Europe’s leadership in regulating digital technologies provides a strong foundation, but even its frameworks require updates to tackle issues like misinformation and bias in AI outputs. Meanwhile, the United States’ innovation-focused approach leaves critical gaps in accountability and user protection.
The integration of binding ethical principles, enhanced algorithmic transparency, and globally coordinated regulations is essential to ensure that AI technologies and search engines evolve responsibly. By addressing these gaps, platforms can foster trust, safeguard user rights, and remain legally compliant in an increasingly complex digital ecosystem.
3. Potential Legal Non-Compliance in AI Systems: Navigating Violated Rights and Liability
Question: With AI systems shaping how information is delivered, what legal risks do platforms face, and how can they ensure compliance while avoiding reputational or economic damages?
Response: The legal framework surrounding AI is evolving rapidly, but at its core, it emphasizes human rights, transparency, and accountability. Violations of these principles can lead to significant civil liability, legal penalties, and damage to trust in AI platforms. Below, we’ll dissect the key areas of legal non-compliance, from violated individual rights to the systemic risks posed by opaque algorithms.
3.1. Violated Rights
AI systems, including search engines and generative platforms, may inadvertently infringe on fundamental rights, whether through incorrect outputs, biased algorithms, or misuse of data. Let’s explore how these rights can be violated and the associated risks.
3.1.1. Right to Honor, Privacy, and Self-Image
Article 18 of the Spanish Constitution guarantees individuals protection over their honor, personal privacy, and image. However, AI platforms frequently risk violating these rights due to:
a. Dissemination of Defamatory or Inaccurate Information
- Risk: AI-generated responses may include false or defamatory statements about individuals or companies, especially when drawing from unverified sources.
- Example: An AI search result falsely claims a CEO was involved in fraud. This harms their reputation, potentially resulting in defamation lawsuits or economic fallout.
- AEO Insight: Ensuring data accuracy is critical. Structured data (e.g.,
ClaimReview
) and authoritative sources must be prioritized to reduce reputational risks.
b. Non-Consensual Use of Personal Data
- Risk: AI systems often scrape the internet for training data, including sensitive personal information, which may have been obtained without consent.
- Example: Photos from social media used in training datasets could infringe on privacy rights if not authorized by the individual.
c. Inability to Rectify False Data
- Risk: When AI platforms propagate false information, affected individuals may face significant barriers to rectification or deletion, violating their constitutional rights.
- Legal Implication: Platforms without clear correction mechanisms may breach the right to privacy and honor, leaving them exposed to legal claims.
Solution:
- Platforms must implement transparent and accessible processes for requesting corrections (e.g., rectifying false data about an individual’s financial status).
- Technical Measure: Employ schema such as
CorrectionComment
to document corrections publicly, boosting transparency and trust.
3.1.2. Right to the Protection of Personal Data and Digital Oblivion
The General Data Protection Regulation (GDPR) and the right to be forgotten grant individuals significant control over their personal data. Yet, AI platforms risk non-compliance in the following ways:
a. Misuse of Personal Data
- Risk: AI models trained on improperly obtained or outdated data may violate GDPR provisions requiring data minimization and accuracy.
- Example: A platform fails to delete an individual’s past criminal record, despite the record being expunged under the right to be forgotten.
b. Lack of Explicit Consent
- Risk: Many AI systems process personal data without obtaining explicit, informed consent, which is a core GDPR requirement.
- Example: Data scraped from blogs or forums is included in training datasets without notifying the data subjects.
c. Difficulty in Data Removal
- Risk: Platforms often lack user-friendly tools to request the removal of specific information, violating Article 17 of the GDPR (Right to Erasure).
- Example: A user who finds their outdated LinkedIn profile repeatedly cited in AI outputs struggles to have the references removed.
Solution:
- User Controls: Provide clear, self-service tools to allow users to request deletions, corrections, or updates.
- AEO Tip: Embed
Person
andAboutPage
schema into user-facing tools to help search engines recognize and prioritize correction requests.
3.1.3. Right to Truthful Information and Equitable Access to Sources
Article 20 of the Spanish Constitution emphasizes citizens’ right to access truthful and impartial information. AI platforms can undermine this right in the following ways:
a. False or Outdated Information
- Risk: Delays in updating databases or errors in algorithmic logic may result in the dissemination of outdated or incorrect information.
- Example: A search engine provides outdated COVID-19 statistics, misinforming users about current public health risks.
- AEO Insight: Use real-time APIs and structured data (e.g.,
dateModified
) to ensure search outputs reflect the most current information.
b. Commercial Prioritization
- Risk: AI platforms may prioritize sponsored content or corporate-aligned narratives, limiting access to objective information.
- Example: A search query on environmental issues favors articles from fossil fuel companies over independent researchers.
c. Lack of Source Diversity
- Risk: Over-reliance on dominant sources can exclude minority perspectives, resulting in a lack of information plurality.
- Example: News coverage that only references major media outlets may omit regional or dissident viewpoints.
Solution:
- Integrate source diversity algorithms to include a wide range of perspectives while prioritizing credibility and authority.
- Use
IsPartOf
schema to connect related content, ensuring balanced representation of sources.
3.2. Civil Liability and Possible Economic and Reputational Damages
AI platforms are not just facilitators of information—they are legally accountable for the consequences of their outputs. Let’s explore two major areas of liability.
3.2.1. Dissemination of False or Biased Information
a. Reputational Damage
- Impact: False or defamatory outputs can ruin an individual’s or company’s public image.
- Example: An AI incorrectly attributes a controversial quote to a public figure, leading to widespread backlash.
- Legal Implication: Victims may sue for defamation, with courts requiring platforms to compensate for reputational harm.
b. Economic Impact
- Impact: Incorrect information about a company’s financial health can result in lost clients, stock market drops, or revenue losses.
- Example: A tech company’s valuation plummets after an AI falsely reports their bankruptcy.
- AEO Insight: Fact-checking workflows (e.g.,
ClaimReview
) can mitigate the spread of false financial claims.
c. Manipulation of Public Opinion
- Impact: Biased or false outputs influence elections, referendums, or social debates, eroding trust in institutions.
- Example: AI-generated misinformation influences voter behavior in a national election.
3.2.2. Unauthorized Use of Personal Data and Protected Content
a. Legal Violations
- Risk: Using copyrighted content or personal data without authorization breaches GDPR and intellectual property laws.
- Example: An AI uses a copyrighted image without licensing, leading to copyright infringement claims.
b. Financial Liability
- Impact: Those affected can sue for monetary damages, particularly for improper data usage or misappropriation of intellectual property.
Solution:
- Use robust licensing agreements for training datasets and ensure all personal data complies with GDPR’s legal basis requirements.
- Implement clear attribution via schema such as
CopyrightHolder
to properly credit protected content.
3.3. Lack of Transparency and Algorithmic Control
Transparency is both a regulatory requirement and a trust-building tool in AEO. Without visibility into how AI systems operate, platforms risk alienating users and inviting legal scrutiny.
3.3.1. Black Box Risks in AI Systems
a. Inability to Audit Decisions
- Risk: Users and regulators cannot question how decisions are made without access to the AI’s internal logic.
- Example: An individual is denied a loan based on an AI assessment but cannot understand why or challenge the outcome.
b. Lack of Accountability
- Risk: Companies often claim ignorance of algorithmic errors due to the complexity of their systems, leaving victims without recourse.
3.3.2. Biases and Manipulation of Search Results
a. Discrimination
- Risk: AI outputs may disproportionately favor or disadvantage certain ethnic groups, genders, or regions due to biased training data.
- Example: Job recommendations skewed toward male candidates over equally qualified female candidates.
b. Priority Manipulation
- Risk: Search results may be manipulated to favor certain commercial or political interests, compromising objectivity.
Conclusion: Building Legal Resilience and Trust
Addressing these risks requires a holistic strategy combining legal compliance, transparent workflows, and AEO-focused optimizations. By prioritizing accuracy, diversity, and user control, platforms can mitigate legal liability while enhancing their credibility in an AI-driven world.
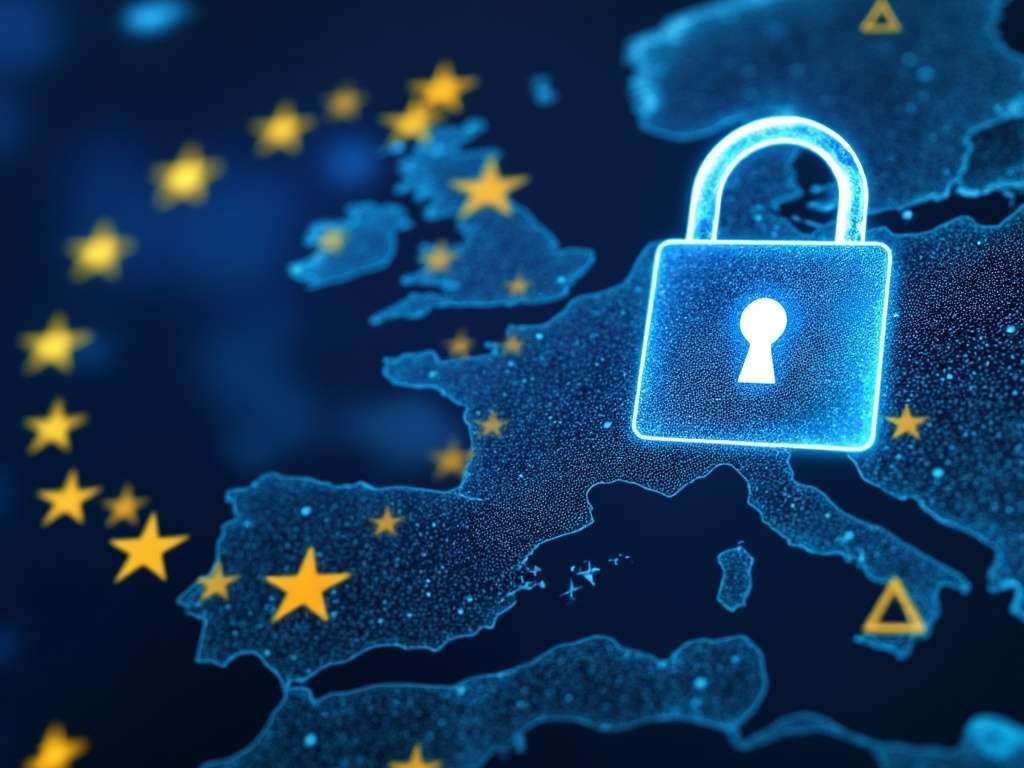
4. Solution Proposals – Regulation of AI in Europe and the US
This chapter details a set of specific proposals designed to address the legal and ethical challenges related to artificial intelligences (AIs) and search engines. These solutions seek to guarantee that the rights of people and organizations are respected, promote the transparency of technological systems and prevent the spread of disinformation.
4.1. Right to exclusion and segmentation of information
The right to granular opt-out allows citizens and organizations to have control over how and where information related to them is used. This right is fundamental in a digital environment where AIs process and present data autonomously, often without direct human supervision.
Specific proposals
4.1.1. Exclusion by data category
Users may request the selective exclusion of information in the following categories:
- Sensitive personal data:
- Information related to health, religion, sexual orientation, criminal record or other aspects protected by the General Data Protection Regulation (GDPR).
- Practical example: An individual may request that medical diagnoses or religious affiliations not be mentioned in AI-generated results, even if such information is available from public sources.
- AI-generated reviews:
- AIs are prohibited from generating subjective judgments or interpretive analyzes about people or organizations.
- Practical example: A company can require that opinions such as “Company X has poor service” not be generated, limiting responses to objective data supported by reliable sources.
- Thematic associations:
- Users can request that their name or reference not be associated with specific topics (e.g., scandals, crimes, political affiliations).
- Practical example: A public figure involved in a past controversy could ask that such an association not be mentioned in current AI-generated results, to protect his or her right to honor and reputation.
4.1.2. Mandatory consent for sensitive topics
The protection of sensitive data requires explicit and renewable consent for its processing and display in AI systems.
- Explicit consent:
- Before processing or displaying data related to sensitive topics, platforms must obtain clear and detailed permission from the affected party.
- This consent must specify the purpose of use and the context in which the information will be applied.
- Periodic renewal:
- Consent must be renewed periodically to ensure its validity, especially if circumstances or the intended use of the data change.
- Prohibition of unauthorized use:
- In the absence of consent, platforms will not be able to process or display sensitive data, even if it is publicly available.
Practical example:
A company may authorize its financial results to be mentioned only in verified reports, prohibiting the inclusion of subjective interpretations of its economic performance.
4.1.3. Clear exclusion deadlines
Establishing defined deadlines for the implementation of exclusion requests guarantees the effectiveness of the right.
- Ordinary request:
- Exclusion requests must be resolved within a maximum period of 30 days from receipt.
- Urgent request:
- In cases where there is imminent reputational damage or a significant risk of misinformation, requests must be processed within a period of no more than 5 business days.
Notification systems:
- Initial and progress notifications:
- Applicants should receive immediate confirmation upon registration of their application, as well as periodic updates on the status of their application.
- Exclusion confirmation:
- Once the exclusion is implemented, the applicant will receive a detailed notification indicating:
- Which platforms have implemented the exclusion.
- What type of information has been removed or restricted.
- Once the exclusion is implemented, the applicant will receive a detailed notification indicating:
Practical example:
A company affected by false rumors on social networks requests the exclusion of thematic associations in search engines. You receive regular notifications confirming the removal of such associations within 3 business days.
Impact and Benefits of the Proposed Regulation
- Protection of the right to honor and privacy:
- People and companies will be able to limit access to sensitive or incorrect information, avoiding reputational damage.
- Control over information:
- Organizations will be able to avoid erroneous or speculative interpretations about their activity, maintaining their credibility with clients and partners.
- Promotion of a trustworthy information environment:
- These measures will force technology platforms to improve the quality and accuracy of their content generation systems.
4.2. Public and secure registry to manage exclusion requests
A public, centralized registry is essential to ensure that opt-out requests are processed uniformly and efficiently by all technology platforms. This registry would serve as a single point of reference for citizens and organizations, ensuring transparency in the management of their rights.
Specific proposals
4.2.1. Unique management system
- Registry layout:
- Create a centralized digital platform, managed by an independent regulatory body (e.g., the Spanish Data Protection Agency in the EU or a similar entity in the US).
- Registration will allow users to:
- Register exclusion requests with detailed specifications.
- Manage and update existing requests.
- Check the status of your implementation.
- System functionalities:
- Easy access: Intuitive and accessible design for all users, regardless of their level of digital literacy.
- Multilingual support: Support for different languages, including those relevant to specific jurisdictions.
- Free: Access to the registry and management of requests must be free to guarantee equal access.
4.2.2. Authentication and security
- Strong authentication:
- Use advanced technologies to verify the identity of applicants, such as:
- Two-factor authentication (2FA).
- Biometric identification (e.g., facial recognition or fingerprint).
- Verification through official documents (e.g., passport, ID).
- Use advanced technologies to verify the identity of applicants, such as:
- Data protection:
- Implement end-to-end encryption systems to ensure applicant information is protected from unauthorized access.
- Ensure that the data stored in the registry is not accessible to third parties for commercial purposes or purposes unrelated to its initial purpose.
- Abuse prevention:
- Establish mechanisms to prevent misuse of the registry, such as false requests or denial of service (DDoS) attacks.
4.2.3. Interoperability
- Connection with platforms:
- Force the main technological platforms (search engines, generative AIs, social networks) to synchronize their systems with the public registry.
- Synchronization will ensure that exclusions are automatically and consistently implemented across all relevant services.
- Common technical standards:
- Establish standard protocols for data transmission between the registry and platforms, ensuring compatibility and accuracy of information.
- Real time update:
- Requests processed in the registry must be reflected in the platforms’ systems within a maximum period of 24 hours, minimizing the impact of the delay in implementation.
4.2.4. History and transparency
- History consultation:
- Users will be able to access a complete history of their requests, which will include:
- Registration date.
- Current status (e.g., pending, in process, completed).
- Confirmation of implementation on each linked platform.
- Users will be able to access a complete history of their requests, which will include:
- Transparency in implementation:
- The system shall generate automatic reports detailing how exclusion has been implemented on each platform.
- In the event of non-compliance, the registry must notify the user and escalate the situation to the competent regulatory body.
- Right to rectification:
- Users may modify or withdraw their requests at any time through the portal, with immediate effect on the linked platforms.
Practical example
A user, affected by the dissemination of incorrect information on various platforms, accesses the registry to manage his or her exclusion:
- Initial request:
- The user details that they wish to exclude their name and certain personal data (e.g., references to a labor scandal) from search engines and generative AIs.
- Complete the authentication using your ID and a verification code sent to your mobile.
- Confirmation of the process:
- You receive a unique tracking number to check the status of your request.
- Interoperability in action:
- Within 24 hours, the registry notifies the user that their exclusion has been implemented in Google, Bing, ChatGPT and Perplexity, among others.
- History query:
- The user accesses the history and confirms that all relevant platforms have removed the requested references.
- Non-compliance notification:
- If any platform does not comply, the registry automatically generates a notice to the regulatory body to investigate and sanction if necessary.
Expected impact
- Efficiency:
- A single registration will streamline the opt-out process, eliminating the need to contact each platform individually.
- Transparency:
- Users will have complete visibility into how and where their requests were implemented.
- Trust:
- The centralization of the system will increase the confidence of citizens and organizations in the ethical and responsible management of their data.
4.3. Mandatory verification of sources and constant updating of data
To combat misinformation and guarantee the quality of the information provided by AIs and search engines, it is essential to implement mechanisms that prioritize the veracity and timeliness of the sources. This approach not only protects end users from incorrect information, but also strengthens trust in these technologies.
Specific proposals
4.3.1. Ranking of reliable sources
To guarantee the reliability of the information used by AIs, platforms must establish a classification system that identifies and prioritizes reliable sources.
- Classification criteria:
- Track record and reputation: Sources must have a verifiable track record of accuracy in their publications. Example: Academic institutions, recognized media, government agencies.
- Editorial rigor: Sources must demonstrate rigorous review processes to validate information before publishing it.
- Transparency: Sources need to clearly indicate the origin of their data and the methodology used to collect it.
- Scoring system:
- Platforms must assign trustworthiness scores to sources, based on the above criteria.
- Sources with low scores will be used with restrictions or completely excluded from the responses generated.
- Public database:
- Create an accessible registry of sources ranked with their ratings, managed by an independent regulatory body.
Practical example:
An AI platform prioritizes information from “The World Bank” over economic statistics due to its high score in editorial rigor and transparency, while excluding personal blogs or forums without validation.
4.3.2. Mandatory labeling
Each AI-generated response must include clear information about the sources used, so that users can evaluate the reliability of the information presented.
- Minimum information required:
- Source name.
- Date of publication of the original content.
- Reliability level assigned to the source.
- Standard labels:
- Include labels that indicate whether the information is recent, potentially outdated, or unverifiable. Example:
- “Updated: data as of [date].”
- “Source with high reliability: [name of source].”
- “Caution: source with limited reliability.”
- Include labels that indicate whether the information is recent, potentially outdated, or unverifiable. Example:
- Accessible design:
- Labels should be easily visible and understandable, even for users with low levels of digital literacy.
Practical example:
An AI’s response about a company includes: “According to [European Central Bank], projected inflation for 2024 is 2.1% (updated 11/15/2024). Source rated highly reliable.”
4.3.3. Real time update
To prevent the spread of outdated information, platforms will need to constantly update their databases and sources.
- Update frequency:
- Platforms must update their databases at least every 24-48 hours to reflect significant changes in public information.
- Outdated content alert:
- In cases where the information has not been recently updated, platforms must include a warning in the responses generated.
- Example: “This information was last updated on [date], it may not reflect the most recent data.”
- Collaboration with sources:
- Platforms should establish agreements with trusted sources to receive real-time updates on critical topics (e.g., economic statistics, medical advances).
Practical example:
If an AI responds to a query about a natural disaster, it prioritizes information from an organization like the Red Cross, updated hourly during emergency situations.
4.3.4. Answers based only on verifiable facts
AIs should limit their responses to information supported by reliable sources, eliminating any speculative or subjective content.
- Prohibition of speculation:
- Responses may not include interpretations or opinions not supported by verifiable evidence.
- Prioritization of facts:
- The generation of responses should focus on documented facts, always citing supporting sources.
- Detection of inconsistencies:
- Platforms will need to implement algorithms to detect and filter inconsistent information across multiple sources before generating a response.
Practical example:
Instead of answering: “Company € in the third quarter of 2024.”
General practical example
A user consults an AI about the financial results of a company in the last quarter. The AI responds:
- “According to [reliable source: National Securities Market Commission], company Source classified as highly reliable.”
- Additional tag: “Data confirmed by a recognized source. This information is current and verified.”
Expected impact
- Greater user confidence:
- Including reliable sources and removing speculative content will strengthen the credibility of AI-generated answers.
- Misinformation reduction:
- A constant classification and verification system will prevent the spread of erroneous or outdated data.
- Information transparency:
- Clear labels will allow users to critically evaluate the quality of the information provided.
4.4. Algorithmic transparency guarantee
Algorithmic transparency is essential to ensure that artificial intelligences (AIs) operate ethically, fairly and in accordance with fundamental rights. This involves making algorithmic processes and decisions visible to prevent bias, ensure accountability, and promote public trust in these technologies.
Specific proposals
4.4.1. Regular audits
Platforms that use AI to generate results or content must submit their systems to periodic independent audits.
- Frequency:
- Conduct audits at least twice a year, or more frequently on high-impact systems (e.g., related to health, finance or security).
- Areas to review:
- Algorithmic biases: Identification of discriminatory patterns in the responses generated by AI.
- Model efficiency: Verification that responses are accurate and based on up-to-date data.
- Regulatory compliance: Ensure that algorithms comply with privacy, anti-discrimination and data protection laws.
- Independent auditors:
- The audits must be carried out by certified and independent entities, with experience in technological ethics and digital law.
- Corrective measures:
- If irregularities are identified, the platforms will be obliged to implement improvements within a specific period (e.g., 90 days).
Practical example:
A search engine uses an AI model that prioritizes sources from certain countries over others without technical justification. The audit identifies this bias and requires changes to ensure equal geographic representation.
4.4.2. Impact reports
Platforms will be required to publish regular reports detailing the impact of their algorithms on users and society at large.
- Report content:
- Prioritization criteria: Explain how sources of information are chosen and ordered.
- Analysis of detected biases: Detail the possible biases identified and the measures taken to correct them.
- Measurable results: Show statistics on the accuracy and relevance of the responses generated.
- Frequency:
- Publish reports every six months, with more frequent updates in case of significant algorithm changes.
- Accessible format:
- Ensure that reports are understandable to the general public, with specific sections for experts and regulators.
- Social impact:
- Analyze how algorithmic decisions affect vulnerable or minority groups, with recommendations to minimize harm.
Practical example:
A semiannual report from a platform details that 70% of the prioritized sources on health issues come from recognized organizations, while 30% are less reliable sources. As a corrective measure, a change in the algorithms is announced to increase the proportion of reliable sources.
4.4.3. Regulatory oversight
An independent regulatory body will oversee compliance with algorithmic transparency regulations and will have the authority to intervene in case of irregularities.
- Functions of the regulatory body:
- Audit review: Analyze the results of the audits carried out by the platforms and validate their compliance.
- Proactive investigation: Conduct random inspections to identify unreported irregularities.
- Claims management: Address complaints from users or companies that consider that an algorithm operates in a biased or discriminatory manner.
- Sanctions:
- Impose significant fines on platforms that fail to comply with transparency standards.
- Require temporary suspension of problematic algorithmic systems until necessary fixes are implemented.
- International collaboration:
- Regulatory bodies from different jurisdictions (e.g., EU and US) will need to coordinate to supervise global platforms.
Practical example:
A regulatory body detects that an AI used for job recruitment discriminates against certain demographic groups. Requires the company to develop an action plan to eliminate bias within 60 days.
4.4.4. Public access
Public access to information about algorithms is key to promoting transparency and citizen scrutiny.
- Basic principles:
- Platforms must publish information about:
- How the algorithms work: Simplified explanations of its logic.
- What data they process: Fonts used and how they are selected.
- What decisions they make: How algorithms prioritize or exclude information.
- Platforms must publish information about:
- Intellectual property protection:
- Public access must not compromise trade secrets, but essential information about the operation of the algorithms must be disclosed.
- Accessible interface:
- The platforms must enable an online portal where users can clearly and easily consult the basic principles of their algorithms.
- Education and resources:
- Include educational sections to help users interpret technical information, encouraging more informed use of the systems.
Practical example:
An AI platform offers a public portal where it explains that its algorithm prioritizes government and scientific sources on medical topics, displaying a list of the sources used and their selection criteria.
Expected impact
- Greater confidence:
- Users will trust platforms more knowing that their algorithms are subject to independent and public oversight.
- Abuse prevention:
- Transparency will make it difficult to use algorithms to manipulate information or prioritize undeclared commercial interests.
- Ethics in development:
- Companies will be incentivized to develop more fair and equitable systems, knowing that they will be under scrutiny.
4.5. Strengthened protection against misinformation and bias
The spread of misinformation and algorithmic biases in artificial intelligence (AI) systems can have serious consequences on public perception, the reputation of individuals and companies, and trust in digital technologies. To counter these risks, it is essential to implement active measures that combine technical tools, human supervision and strict sanctions.
Specific proposals
4.5.1. Hybrid detection systems
Hybrid systems combine advanced AI technologies with human monitoring to identify false, biased or unverifiable content before it is spread.
- Initial automatic detection:
- Implement algorithms designed to:
- Identify discrepancies between multiple sources on the same topic.
- Spot common signs of misinformation, such as exaggerated headlines, lack of attribution to reliable sources, or inconsistent data.
- Analyze the reliability history of the sources used.
- Implement algorithms designed to:
- Human supervision:
- Establish teams of specialized moderators who manually review:
- Responses generated on especially sensitive topics (e.g., health, politics, security).
- Content flagged as dubious by users or detected by algorithms.
- Establish teams of specialized moderators who manually review:
- Continuous training:
- Provide ongoing training to human moderators to identify new misinformation tactics and emerging biases in responses.
Practical example:
An AI that generates answers about vaccination automatically analyzes whether the cited sources are supported by recognized health organizations. If a questionable source is detected, the response is flagged for human review before being displayed.
4.5.2. Proactive alerts
When it is not possible to completely verify the information or when there are doubts about its veracity, platforms must include visible alerts in the generated responses.
- Warning labels:
- Answers should include clear labels when:
- The information has not been confirmed by reliable sources.
- The data is old and has not been recently updated.
- There are divided opinions among the cited sources.
- Example of labels:
- “Warning: This information has not been fully verified.”
- “Note: The data used in this answer was last updated on [date].”
- Answers should include clear labels when:
- Options for users:
- Offer users the possibility of:
- Report suspicious or incorrect information.
- Consult directly the original sources used in the answer.
- Offer users the possibility of:
- Update notifications:
- Platforms must alert users if a previously generated response is subsequently corrected or updated with more accurate data.
Practical example:
A user who inquires about an ongoing event receives a response labeled: “Warning: Information about this event is evolving and may not be complete. Please check for more recent updates.”
4.5.3. Penalties
Technological platforms that allow the repeated dissemination of disinformation or biased content must face sanctions proportional to the severity and scope of the damage caused.
- Financial fines:
- Financial sanctions must be significant to deter negligent practices.
- Example: Fines calculated based on the scope of the false content (e.g., number of exposed users) and the severity of the damage caused (e.g., reputational impact, economic losses).
- Rectification obligations:
- Platforms must publicly correct incorrect responses generated by their systems, clearly indicating:
- The nature of the error.
- The measures taken to prevent it from happening again.
- Platforms must publicly correct incorrect responses generated by their systems, clearly indicating:
- Suspension of services:
- In serious cases of non-compliance, authorities may order the temporary suspension of AI services or search engines until corrective measures are implemented.
- Intensive supervision:
- Platforms repeatedly sanctioned will be subject to stricter oversight by regulatory bodies.
Practical example:
A search engine that repeatedly generates answers based on outdated data on medical topics is fined, along with an obligation to publicly rectify erroneous answers on its official communication channels.
Expected impact
Misinformation reduction:
Hybrid systems and proactive alerts will, therefore, minimize the spread of false or unverified content, particularly on sensitive topics. In addition, these systems will encourage users to rely on verified information.
Public trust:
Users will, as a result, trust platforms more that implement visible and effective mechanisms to ensure the accuracy of their responses. Furthermore, this increased trust will foster a more reliable digital environment.
Accountability:
Financial sanctions and rectification obligations will, consequently, incentivize platforms to invest in more robust and responsible systems. Moreover, these measures will establish a standard of responsibility that others are likely to follow.
4.6. Regulation of opinions generated by AI
The ability of artificial intelligences (AIs) to issue subjective opinions or judgments, however, poses significant risks, as these responses are often perceived as objective, even though they may lack verifiable support. Moreover, opinions generated by AIs can have serious consequences, including but not limited to reputational damage, information confusion, and manipulation of public perception. As a result, it becomes crucial to address these potential pitfalls. For instance, unchecked AI opinions could lead to widespread misinformation. Therefore, understanding these risks is essential for mitigating their impact. This section establishes measures to regulate this problem effectively.
Specific proposals
4.6.1. Prohibition of opinions
The responses generated by AIs must be limited to verifiable facts and supported by reliable sources, eliminating any subjective component or value judgment.
- Responses limited to facts:
- AIs will be obliged to provide only information based on objective data, always indicating the supporting source.
- Allowed example: “According to [source], company X increased its revenue by 15% this year.”
- Prohibited example: “Company X is doing excellent work in the technology sector.”
- Prohibition of speculative analysis:
- AIs will not be able to generate responses that imply a judgment or interpretation about events, people or organizations, unless they explicitly cite recognized third-party analysis.
- Controlled exceptions:
- In the case of questions that involve opinions (e.g., “Which book is better, X or Y?”), the answer should be limited to:
- Mention perspectives based on recognized surveys or analysis.
- Inform the user that the response does not represent the AI’s own judgment.
- In the case of questions that involve opinions (e.g., “Which book is better, X or Y?”), the answer should be limited to:
4.6.2. Mandatory labeling
Where responses include interpretive analysis or summarize third-party judgments, they should be clearly labeled so that users understand their nature.
- Standard labels:
- Include clear indicators in the responses that inform the user if the information is:
- Based on facts.
- An interpretation.
- A summary of third party opinions.
- Example of labels:
- “Data supported by [source].”
- “Opinion based on analysis of [organization].”
- “This response is a summary of multiple perspectives and does not represent fact.”
- Include clear indicators in the responses that inform the user if the information is:
- Visual differentiation:
- Use visual elements (colors, icons, highlights) to clearly differentiate responses based on facts from those that contain interpretations.
- Automatic tag update:
- Labels should be adjusted automatically if the information presented is subsequently updated or corrected.
Practical example:
A user asks about the best tech companies, and the AI answers: “According to [TechWorld Ranking 2024], company X is ranked first. This is an opinion based on your analysis.”
4.6.3. Right to exclusion against opinions
Individuals and organizations should have the right to completely opt out of any subjective judgment or analysis generated by AIs.
- Opt-out options:
- Allow requests for:
- Prohibit AI from generating opinions about the person or entity.
- Limit responses exclusively to verifiable facts and quotes from third parties.
- Allow requests for:
- Custom implementation:
- Exclusions may be applied on a granular basis, allowing a company to request that opinions not be issued on certain aspects (e.g., its reputation) while allowing objective references to its financial performance.
- Exclusion process:
- Requests must be processed within the established deadlines (e.g., 30 days for general requests, 5 days for urgent cases).
- Users will receive detailed confirmations once exclusions are implemented.
Practical example:
A charity requests that AIs not generate opinions about their social impact, only allowing responses based on audit reports published by verified sources.
4.6.4. Sanctions
Platforms that generate opinions that are inappropriate or without verifiable support must face sanctions proportional to the impact of such responses.
- Financial fines:
- Penalties based on the severity of the damage caused by incorrect or unfounded opinions.
- Example: A scalable fine that considers the scope of the dissemination and the economic or reputational impact on the affected party.
- Obligation to rectify:
- The platforms must:
- Post visible corrections for inappropriate answers.
- Notify users who interacted with the original response about the correction made.
- The platforms must:
- Suspension of features:
- In serious or repeated cases, regulatory authorities may order the suspension of a platform’s ability to generate opinionated content.
- Intensive evaluation:
- Sanctioned platforms will be required to undergo intensive audits to ensure they have corrected identified issues.
Practical example:
An AI repeatedly generates false opinions about a company’s financial performance, which affects its reputation in the market. The platform receives a financial fine and the obligation to publicly rectify the responses, citing verified sources.
Expected impact
- Greater information precision:
- By eliminating unverifiable opinions, the responses generated by AIs will be more objective and reliable.
- Reputational protection:
- People and organizations will be able to avoid harm caused by automatically generated subjective judgments.
- Trust in AIs:
- The implementation of these measures will reinforce the perception that AIs are responsible and ethical tools.
4.7. Educational campaigns on safe and critical use of AI-based technologies
Digital literacy is essential to prepare citizens for the risks and opportunities associated with technologies based on artificial intelligence (AI). Education must be inclusive and accessible, with the aim of promoting critical thinking, protecting digital rights and empowering users to take advantage of these tools responsibly.
Specific proposals
4.7.1. Programs in educational institutions
Integrate teaching about the ethical, safe and critical use of AI-based technologies into the curricula of schools, colleges and universities.
- Updated curricula:
- Include specific modules on AI in subjects such as technology, social sciences and ethics.
- Key contents:
- How AIs work and their limitations.
- Identification of misinformation and algorithmic biases.
- Digital rights, such as the right to privacy and data protection.
- Practical workshops:
- Carry out interactive activities where students can:
- Analyze real examples of AI-generated disinformation.
- Evaluate the reliability of online information sources.
- Simulate scenarios where they exercise their right to digital exclusion.
- Carry out interactive activities where students can:
- Teacher training:
- Train teachers in the safe and critical use of digital technologies so they can teach these concepts effectively.
Practical example:
A technology module in high school includes an exercise where students use an AI tool to generate content and then analyze how sources were selected and what biases might be present.
4.7.2. Public campaigns
Launch information campaigns in traditional and digital media to raise public awareness about the risks and benefits of AI, and how to use them responsibly.
- Aim:
- Increase awareness about:
- The impact of AI-generated responses.
- The importance of verifying the information presented.
- How to exercise rights such as exclusion or digital rectification.
- Increase awareness about:
- Formats:
- Television and radio spots: Briefly and clearly explain the risks associated with AIs and the tools available to protect yourself.
- Social networks: Publish educational content with practical examples and easy-to-implement tips.
- Local events: Organize community talks and workshops aimed at different age groups.
- Collaborations:
- Partner with technology platforms, NGOs and governments to maximize the reach of campaigns.
Practical example:
A social media ad shows how a user can verify the authenticity of an AI-generated response, with a link to additional resources to learn more.
4.7.3. Accessible resources
Develop free educational tools and materials that enable anyone to learn to safely and critically interact with AI-based technologies.
- Digital libraries:
- Create an online repository with resources such as:
- Practical guides on how AIs work.
- Tutorials to identify misinformation.
- Tips on how to exercise digital rights.
- Create an online repository with resources such as:
- Educational applications:
- Develop apps that offer:
- Interactive simulations on how AIs generate content.
- Educational games to teach how to detect bias or data manipulation.
- Develop apps that offer:
- Digital inclusion:
- Ensure that resources are accessible to people with disabilities (e.g., braille versions, audio narrations).
- Translate materials into multiple languages to reach diverse communities.
Practical example:
A government website offers a free AI literacy course, including explanatory videos, practical exercises, and a digital rights FAQ section.
Expected impact
- Increased public awareness:
- Citizens will be better prepared to identify misinformation and protect themselves against the risks associated with AI.
- Inclusive training:
- Educational campaigns will reach a wide variety of audiences, promoting equality in access to digital knowledge.
- Trust in technology:
- A well-informed citizenry will have a more critical and balanced relationship with AI, taking advantage of its benefits while minimizing the risks.
5. Technical Implementation of the Proposed Solutions – Regulation of AI in Europe and the US
This chapter outlines how to practically implement the measures described in the previous section. Specifically, it provides the technical and organizational steps necessary to ensure the seamless integration of exclusion mechanisms, information verification, and algorithmic transparency in search engines and AI systems. By following these steps, stakeholders can better align technological functionality with ethical and legal standards.
5.1. Integration of Exclusion Mechanisms in Search Engines and AIs
To begin with, integrating opt-out mechanisms is crucial for ensuring that users and organizations can exercise their right to remain excluded from results generated by AI or search engines. This process not only reinforces individual rights but also enhances trust in these technologies.
Adaptation of Existing Systems
First and foremost, search engines and AI platforms must adapt their existing systems to accommodate exclusion requests. To do this, they need to incorporate specific functionalities such as:
- Exclusion Databases: These are centralized and synchronized lists containing active opt-out requests. Importantly, they must be accessible to all relevant platforms, ensuring consistency across the digital ecosystem.
- Automated Filters: These algorithms play a key role in identifying and blocking the generation of responses related to the terms specified in exclusion requests. By automating this process, platforms can handle opt-outs efficiently and accurately.
Synchronization with Public Records
Furthermore, platforms will need to establish robust synchronization with the public registry described in Chapter 4.2. This ensures that opt-out requests are implemented uniformly and without duplication of effort across multiple systems. Consequently, this step reduces redundancy and enhances user satisfaction.
Integration Tests
Moreover, before launching these mechanisms, platforms must conduct rigorous integration testing. These tests serve multiple purposes, such as ensuring:
- Correct Application of Exclusions: Platforms must verify that exclusions are applied precisely across all systems without error.
- Preservation of System Performance: Testing should confirm that these mechanisms do not negatively affect the overall functionality or speed of the AI-generated responses.
Practical Example
To illustrate, consider the case of a user who requests that their name be excluded from all responses related to a past medical incident. This request is entered into the public registry and, through automatic synchronization, is successfully blocked on platforms like Google, ChatGPT, and Perplexity within 24 hours. This seamless execution demonstrates the effectiveness of integrated exclusion mechanisms.
Key Transition Benefits
In conclusion, integrating exclusion mechanisms is not just a technical necessity but also a foundational step toward ensuring ethical compliance and enhancing user trust. Additionally, by adapting existing systems, synchronizing with public records, and conducting thorough integration tests, platforms can create a more transparent and responsible AI ecosystem.
5.2. Fast Protocols for Processing and Resolving Requests: A Legal and AEO-Optimized Approach
In a digital ecosystem where AI-generated information is dynamic and subject to rapid dissemination, how can platforms effectively manage and resolve data exclusion, correction, or update requests without compromising legal compliance or user trust?
Fast and structured protocols are not just a best practice—they’re a legal and operational necessity. Delays in resolving requests related to incorrect or outdated information can lead to reputational harm, regulatory penalties, and erosion of user trust. To tackle this, platforms must implement expedited workflows supported by automation, human oversight, and clear communication. Let’s break this down.
Why Speed and Precision Matter
When AI platforms distribute content—whether search-generated or query-specific—it carries inherent legal and reputational risks. Errors in data (e.g., a company’s financial metrics or individual professional details) can quickly spiral into lawsuits, particularly under consumer protection laws, GDPR, or defamation statutes. Additionally, slow processing undermines Answer Engine Optimization (AEO), as trust signals like timely updates and responsiveness heavily influence user satisfaction and search engine trust rankings.
Expedited resolution protocols bridge this gap by meeting both legal mandates and user expectations for efficient and transparent processes.
Specific Deadlines for Request Processing
Establishing clear deadlines for resolving requests helps platforms maintain compliance and reduce legal risks while fostering trust. Here’s how timelines can be structured:
1. Standard Processing
- Timeframe: Resolve general requests within 30 calendar days, a standard compliant with frameworks like the GDPR (Article 12) for user data correction or erasure.
- Example Requests: Minor factual inaccuracies, outdated source data, or low-risk exclusion requests.
- AEO Tip: Meeting this standard reinforces transparency and accountability, which are key trust factors in AI-driven user experiences.
2. Urgent Processing
- Timeframe: Handle requests with immediate reputational or financial implications in 5 business days or less. This includes cases where data errors could lead to significant harm (e.g., misinformation about an ongoing lawsuit, financial misrepresentation, or health-related inaccuracies).
- Legal Tie-In: Many jurisdictions recognize the urgency of “critical harm” scenarios, where undue delays could constitute negligence.
Balancing Efficiency and Accuracy
While expedited timelines are essential, platforms must not compromise on accuracy. Legal compliance hinges on resolving requests in a manner that is both timely and substantively thorough.
Workflow Automation for Request Processing
To manage requests efficiently and at scale, AI platforms need robust automation tools. These systems streamline request intake, tracking, and resolution while minimizing human error. Key steps include:
1. Automated Request Registration
- AI-driven intake forms categorize requests (e.g., data correction, exclusion, or update) and forward them to the appropriate department automatically.
- AEO Insight: Incorporate schema markup (e.g.,
Action
types likeUpdateAction
orDeleteAction
) into platform forms to enhance structured tracking and compliance documentation.
2. Real-Time Notifications
- Notify the requester at every stage of the process:
- Acknowledgment: Confirm receipt of the request within minutes.
- Updates: Provide status updates (e.g., “Request under review” or “Awaiting documentation”).
- Resolution: Share final outcomes, including a summary of actions taken.
- Legal Benefit: Transparent communication reduces the likelihood of regulatory complaints or escalation.
3. Resolution Confirmation and Audit Trail
- After resolving a request, the system should:
- Provide a detailed report of changes made.
- Log the resolution in an immutable audit trail for future reference.
- Share a downloadable record with the applicant for transparency.
- AEO Impact: An auditable history boosts trustworthiness in search engines that prioritize E-E-A-T (Experience, Expertise, Authority, Trust).
Human Supervision for Complex Cases
While automation is invaluable for efficiency, human oversight remains critical for nuanced or high-risk scenarios. Platforms should maintain specialized teams to:
1. Manage Complex or Ambiguous Cases
- Examples include:
- Requests involving multiple jurisdictions with conflicting legal requirements (e.g., GDPR in the EU vs. CCPA in California).
- Situations where the correction could alter public perception of significant events (e.g., election data).
- Legal Precision: Human teams can ensure compliance with intricate regulatory frameworks and mitigate the risks of automated errors.
2. Conduct Additional Reviews
- In cases where requests are denied or require subjective judgment, a manual review should validate the decision.
- Example: A rejected request to remove a factual claim from a government report must be reviewed to confirm its alignment with public interest and legal standards.
Transparent Processes for Rejected Applications
Rejections, if not handled properly, can trigger user dissatisfaction, appeals, or even lawsuits. A legally sound and user-focused rejection protocol should include:
1. Clear Justifications
- Provide the applicant with a detailed explanation for the rejection, including:
- Reasoning: Why the request does not meet platform guidelines (e.g., the information is accurate or serves the public interest).
- Supporting Evidence: Cite authoritative sources that validate the decision.
2. Appeals Mechanism
- Offer users a channel to contest the decision:
- Example: An appeal form reviewed by a separate team for impartiality.
- Legal Alignment: GDPR and similar laws mandate the right to contest decisions affecting personal data or reputational interests.
Practical Example: Resolving a Data Correction Request
Scenario: A company discovers that an AI-generated response falsely states its annual revenue as $15 million, rather than the accurate figure of $50 million. This misinformation risks harming the company’s reputation among investors and clients.
Workflow for Resolution:
- Request Submission: The company submits a correction request via an automated portal.
- Urgent Processing Flagged: The system categorizes the request as “urgent” due to its potential reputational impact.
- Immediate Action: Within 24 hours, the AI system:
- Locks the incorrect response from public display.
- Reviews and verifies the accurate data using reliable sources (e.g., the company’s audited financial statements or filings).
- Notification: The platform notifies the company of the resolution, providing:
- A summary of actions taken.
- A downloadable report with corrected details and timestamps for compliance purposes.
Outcome: The company’s reputation is safeguarded, while the platform demonstrates accountability and legal compliance.
5.2.1 AEO Impact of Fast Request Protocols
Fast and efficient resolution of data correction or exclusion requests directly enhances AEO in multiple ways:
1. Strengthening Trust
- Search engines increasingly prioritize trust signals when ranking AI-generated content. Platforms that handle data accurately and transparently establish themselves as credible information providers.
2. Minimizing Negative Sentiment
- Delays in correcting errors can lead to public backlash or bad press, negatively impacting user perceptions and engagement metrics.
3. Meeting Legal and Ethical Standards
- By aligning with GDPR, CCPA, and similar regulations, platforms avoid penalties while fostering user confidence, a critical driver of long-term success in AI-driven ecosystems.
Fast protocols for processing and resolving requests are the linchpin of legally compliant and user-focused AI systems. By combining automation, human oversight, and transparent processes, platforms can resolve requests efficiently while protecting their reputations and meeting regulatory standards. From automated workflows to specialized review teams, each component plays a vital role in building a reliable and trusted AI environment. Ultimately, a fast, fair, and accurate resolution process is not just a compliance measure—it’s a competitive edge in the ever-evolving landscape of AEO.
5.3. Automated systems to verify and update sources
1. Intelligent Crawlers for Source Management
Automated crawlers are essentially the AI’s investigative reporters, scouring the web for updates. But how can these tools ensure relevance and credibility?
a. Identifying Updates to Existing Sources
AI systems should actively monitor pre-approved sources for updates. For instance:
- Example: If Spain’s National Institute of Statistics (INE) revises its unemployment data, the AI should detect and refresh this information instantly.
- AEO Insight: Always prioritize high-authority sources like government websites or peer-reviewed journals, as search engines and users alike trust these implicitly.
b. Detecting Source Inconsistencies
Imagine one source reports inflation at 3% while another claims 5%. How should the AI resolve this?
- Use source scoring algorithms that weigh reliability factors like authority, historical accuracy, and cross-verification.
- AEO Tip: Schema markup (e.g.,
SameAs
orCitation
) can highlight corroborated data points to improve AI-driven consistency checks.
2. Legal Safeguards for Automated Systems
While technical accuracy is critical, so is staying legally compliant. But what risks are AI systems most vulnerable to?
a. Consumer Protection Laws
If AI outputs outdated or inaccurate data, it could mislead users, leading to lawsuits under false advertising or negligence claims.
- Solution: Include clear disclaimers such as, “Data last updated on [date].” This builds transparency and mitigates risk.
b. GDPR and Data Accuracy
Under the GDPR, data controllers (including AI systems) must ensure information accuracy, especially if it impacts user rights.
- AEO Insight: Use real-time monitoring to automatically update sensitive data or flag inaccuracies as required by compliance frameworks.
3. Automated Reliability Assessment: From Algorithms to Ethics
How does the AI evaluate which sources to trust, and how can it prevent bias or legal challenges?
a. Evaluation Algorithms
Set clear criteria for assessing a source’s credibility:
- Editorial Rigor: Does the source adhere to academic or journalistic standards?
- Track Record: Does this source have a history of reliability?
- Transparency: Are the authors clearly identified and reputable?
b. Dynamic Scoring Systems
Implement a reliability score for each source, recalibrated as performance changes.
- Example: A government agency may maintain a high score, while a news site with multiple corrections may fluctuate.
Legal Relevance: This objectivity safeguards the AI provider against bias or defamation claims, as these assessments can be transparently documented and defended.
4. Handling Outdated Information: Legal and AEO-Driven Strategies
Outdated data isn’t just an annoyance—it’s a legal and trust-breaking liability. So, what’s the solution?
a. Real-Time Monitoring
Define update thresholds based on industry needs:
- Example: Public health data might require daily updates, while historical data may only need annual checks.
b. User Warnings
If data is older than its industry-defined threshold, notify users with clear language:
- Example Warning: “This unemployment statistic was last updated 60 days ago. For the most recent data, please check the source directly.”
AEO Tip: Integrating real-time timestamps using schema properties like dateModified
or datePublished
enhances trust signals to users and search engines.
5. Collaborating with Reliable Sources: Building Trust Pipelines
No AI system operates in a vacuum. Partnering with authoritative organizations ensures continuous access to verified data streams.
a. Partnering with Trusted Institutions
Examples include:
- Government Agencies: Access APIs for official updates (e.g., U.S. Census Bureau or WHO).
- Professional Associations: Collaborate with bar associations or scientific organizations for niche data.
b. Real-Time Data Integration
APIs from these partners allow the AI to pull updates directly into its knowledge base, minimizing lag between real-world changes and AI outputs.
Legal Considerations:
- Comply with data-sharing agreements.
- Avoid exclusivity deals that could violate anti-competition laws.
Practical Example: AI in Legal and Economic Queries
Let’s break down a real-world scenario:
User Query: “What is the current unemployment rate in Spain?”
AI Workflow:
- Source Verification: The AI crawls Spain’s INE website for the most recent statistics.
- Reliability Check: The INE’s dynamic score confirms its authority and transparency.
- Timeliness Validation: The data was updated within the past week, ensuring relevance.
- Delivery: The user receives the latest unemployment rate, a citation, and a timestamp for accuracy.
Why it Matters: This not only satisfies the user but also builds trust by demonstrating legal compliance and ethical transparency.
6. Bridging Technical and Legal: Why It’s Key for AEO
Automated systems must go beyond retrieving data—they must integrate legal safeguards into their workflows. Why? Because modern users (and search engines) are hyper-aware of credibility signals.
- Technical Mastery: By embedding schema, real-time monitoring, and source scoring, AI systems gain trust from AI-driven engines like Google’s SGE (Search Generative Experience).
- Legal Precision: Meeting standards for data accuracy, transparency, and fairness protects against both user mistrust and legal challenges.
Conclusion: A Win-Win for AI Providers and Users
By automating source verification with precision, AI systems achieve more than technical accuracy—they establish themselves as legally compliant, user-first platforms. Whether through intelligent crawlers, real-time updates, or legal safeguards, these strategies inspire trust while meeting the highest ethical standards.
5.4. Minimum Transparency and Traceability Requirements in Algorithms
Building trust in artificial intelligence (AI) systems requires unwavering dedication to transparency and traceability. These principles not only foster user confidence but also safeguard digital rights and encourage ethical AI usage. Transparency means providing clear explanations of how algorithms work, while traceability ensures every decision can be tracked and reviewed. Together, these pillars address concerns about fairness, accountability, and ethical AI deployment.
Internal Records of Algorithmic Decisions
AI platforms must establish robust systems to internally document every algorithmic decision. Maintaining these records can greatly enhance accountability and provide a basis for addressing user concerns. Key elements include:
- Sources Used: Platforms should log all sources that inform algorithmic outputs, detailing their origins and reliability.
- Logical Steps: A clear record of the decision-making process, from computations to inferences, ensures transparency in how conclusions are drawn.
- Filters Applied: Any modifications, such as excluded data or prioritized sources, should be transparently noted to capture the full decision context.
These logs are indispensable for audits and dispute resolution, offering clarity and accountability while upholding ethical standards.
Public Query Interface
To ensure end users have access to relevant insights, platforms must provide a user-friendly public query interface. This tool empowers users to:
- Trace Responses: See the step-by-step process behind an algorithm’s output, including the sources and data used.
- Review Prioritization Criteria: Gain clarity on how sources are ranked, weighted, or prioritized, offering insights into any biases present.
Such transparency tools must be simple enough for non-technical users to understand yet comprehensive enough to satisfy more informed audiences.
Transparency Reports
Regular publication of transparency reports reflects a platform’s commitment to ethical operations. These reports should cover:
- Source Reliability Metrics: Highlighting the proportion of responses based on high-quality sources versus less credible ones.
- Exclusion Requests Processed: Summarizing how many requests were received, approved, or denied, accompanied by anonymized examples.
- Algorithmic Changes: Explaining significant updates to algorithms, including their rationale and anticipated effects on users.
Transparency reports act as a public accountability measure, showcasing an organization’s dedication to ethical practices.
Monitored Access for Independent Auditors
Independent auditors play a critical role in verifying the fairness and compliance of algorithms. With monitored access to internal systems, they can assess:
- Fairness Audits: Reviewing outputs to ensure they align with principles of non-discrimination.
- Bias Detection: Identifying and addressing biases in training data or logic.
- Regulatory Compliance: Confirming adherence to laws and guidelines, such as those protecting data privacy.
These audits must allow recommendations for improvement, creating a continuous feedback loop that ensures algorithms evolve responsibly.
Practical Application Example
Imagine a user questioning the accuracy of an AI-generated response citing conflicting sources. With the public query interface, they trace the response to two credible origins, confirming no data was excluded. This transparency allows them to verify the output’s objectivity.
Simultaneously, an independent audit might reveal the algorithm’s preference for government sources over academic ones, prompting recommendations to balance these inputs and improve overall fairness.
Expected Impact
Implementing minimum standards for transparency and traceability can lead to transformative outcomes:
- Faster Request Processing: Platforms can address user queries about corrections or exclusions more efficiently.
- Improved Accuracy: Automated systems for updating and verifying information will enhance the reliability of outputs.
- Strengthened Public Trust: By prioritizing transparency, platforms solidify their reputation as ethical and trustworthy technology providers.
“In my opinion” adopting transparency and traceability measures is both a regulatory obligation and a strategic advantage. These steps will help create a fairer, safer digital ecosystem that aligns AI platforms with societal values, fostering trust and accountability across the board.
6. Conclusions and Call to Action – Regulation of AI in Europe and the US
The rapid evolution of technologies powered by artificial intelligence (AI) and search engines has dramatically reshaped how we access information. While these advancements have unlocked unparalleled opportunities, they have also revealed significant risks to the digital rights of individuals and organizations. This chapter highlights the key issues discussed in this document and calls for immediate action to update regulatory frameworks, safeguard digital rights, and foster a more ethical and trustworthy information environment.
6.1. The Need to Update Regulatory Frameworks in Europe and the United States
As technology progresses, it has become increasingly clear that current laws are insufficient to address the challenges posed by generative AIs and advanced search engines.
Inadequacy of Current Laws
In Europe, frameworks such as the General Data Protection Regulation (GDPR) and the Digital Services Act (DSA) offer a strong starting point for protecting digital rights. However, these laws fail to address emerging issues, including unverified AI-generated opinions and the inherent biases embedded in algorithms.
Similarly, in the United States, Section 230 of the Communications Decency Act shields platforms from liability for user-generated content. While this legal protection has encouraged innovation, it has also created a gap in accountability for the spread of misinformation and bias through AI systems.
Priority Areas for Regulation
To close these gaps, it is essential to focus on:
- Algorithmic Transparency: Ensuring that AI systems are auditable and their decision-making processes are traceable.
- Real-Time Mechanisms: Establishing systems for the exclusion, correction, and rectification of inaccurate or harmful information.
- Accountability for Misinformation: Penalizing platforms that repeatedly disseminate false or biased content.
The Importance of International Coordination
Given the global reach of AI technologies, collaboration between Europe and the United States is imperative. Harmonizing standards across these regions would help ensure fairness, transparency, and ethical practices on a worldwide scale.
6.2. Benefits of Protecting Citizens’ Digital Rights
Updating regulatory frameworks isn’t just about addressing current challenges; it’s also about creating tangible benefits for users and society.
Strengthening Trust
When users feel they have control over how their data is handled and how they are represented in digital systems, trust in technology naturally grows. This trust fosters stronger relationships between users and platforms.
Reducing Reputational and Economic Damage
Measures such as the swift removal of misinformation or the regulation of AI-generated opinions will significantly reduce the personal and financial harm experienced by individuals and businesses alike.
Promoting Equity
Appropriate regulation can ensure that AI systems operate in a way that benefits society as a whole. By preventing harmful biases and prioritizing fairness over commercial interests, these systems can contribute to a more equitable digital environment.
Empowering Citizens Through Education
Educational campaigns will go beyond protecting users from misinformation. They will equip individuals with the skills to critically evaluate the technologies they use, empowering them to make informed decisions in an increasingly complex digital landscape.
6.3. Concrete Proposals for Legislators, Experts, and Civil Society
To ensure meaningful progress, different stakeholders must take targeted actions within their areas of influence.
For Legislators
Lawmakers should create legal frameworks that explicitly regulate generative AIs. This includes:
- Banning unverifiable AI-generated opinions.
- Mandating algorithmic transparency and independent audits.
- Enforcing strict penalties for platforms that fail to comply with these regulations.
For Technology Experts
Industry professionals must focus on developing tools and systems that:
- Enable real-time verification of information sources.
- Simplify the exclusion and rectification of harmful content.
- Reduce algorithmic biases while promoting fairness and inclusivity in AI-generated responses.
For Civil Society
Active involvement from civil society is crucial. This includes:
- Promoting public debates about the ethical and legal implications of AI technologies.
- Demanding higher accountability standards from technology companies.
- Participating in educational initiatives that improve digital literacy and critical thinking skills.
6.4. Final Reflection: Toward an Ethical and Trustworthy Information Environment
The implementation of the proposals outlined in this document represents a significant step toward addressing the technical and legal challenges posed by AI technologies. More importantly, it lays the foundation for a fairer, more ethical, and trustworthy digital ecosystem.
The Path Forward
The transformation of the digital environment cannot be achieved by a single entity. Instead, it requires coordinated efforts among governments, technology companies, and citizens. By working together, we can ensure that technological advancements uphold fundamental rights and prioritize the well-being of society.
A Commitment to the Future
As technologies continue to advance, so too must our laws, ethical principles, and educational resources. Building an equitable digital future is not just a task for policymakers or industry leaders—it is a shared responsibility that belongs to all of us.
Appendices and Annexes
This section presents additional resources and materials that provide clarity on the key terms, legal references and practical examples mentioned in the document. It also includes methodological tools and final reflections to delve deeper into the relationship between digital rights and artificial intelligence (AI), as well as their impact on search engines.
Appendix 1: Glossary of relevant terms
Artificial Intelligence (AI):
Technology that uses advanced algorithms to perform tasks that typically require human intelligence, such as learning, reasoning, and natural language generation.
Search engines:
Digital tools that allow users to search for information on the web using keywords. Modern search engines, powered by AI, generate complete answers instead of just listing links.
Response engines and generative engines:
- Response engines: Systems that provide direct answers to user queries by extracting information from existing sources. For example, a search engine that directly displays the current temperature of a city when asked “What is the weather in Madrid?”
- Generative engines: AI-based systems that generate new content in response to input. They use advanced language models to create text, images or even code that did not previously exist. An example is ChatGPT, which can compose an essay or have a coherent conversation with the user.
Granular exclusion:
Right that allows people and organizations to decide what specific data about them can be processed or displayed by search engines and AI, adjusting to particular contexts or categories.
Disinformation:
False, inaccurate or misleading information distributed intentionally or by mistake, with the potential to cause confusion, manipulation or social harm.
Algorithmic biases:
Distortions or biases in the results generated by algorithms, caused by limitations in the data used for training, model design decisions, or prioritization rules.
Algorithmic traceability:
Ability to track and document decisions made by an algorithmic system, from input data to results, to ensure transparency and accountability.
Appendix 2: Key legal references in Europe and the United States
Europe
- General Data Protection Regulation (GDPR):
Main European regulation that protects personal data and regulates its processing by companies and technology platforms.
Full text: https://eur-lex.europa.eu/eli/reg/2016/679/oj - Digital Services Act (DSA):
Law that regulates content and transparency on digital platforms in the EU, including the obligations of search engines and AI.
Full text: https://eur-lex.europa.eu/eli/reg/2022/2065/oj - Artificial Intelligence Regulation (proposal):
Proposed regulatory framework for AI in the EU, addressing risks and setting requirements for AI systems.
Information: https://digital-strategy.ec.europa.eu/en/policies/european-approach-artificial-intelligence
USA
- Section 230 of the Communications Decency Act:
Protects technology platforms from liability for content generated by third parties.
Full text: https://www.law.cornell.edu/uscode/text/47/230 - California Consumer Privacy Act (CCPA):
Law that gives California residents rights over their personal data, similar to GDPR in Europe.
Full text: https://oag.ca.gov/privacy/ccpa - Illinois Biometric Data Privacy Act (BIPA):
Regulates the collection and storage of biometric data by companies.
Full text: https://www.ilga.gov/legislation/ilcs/ilcs3.asp?ActID=3004
Appendix 3: Additional resources on digital rights and their relationship to AI
To better understand the impact of AI on digital rights, the following tools and methodological studies stand out:
- Global Responsible Artificial Intelligence Index (GPAI):
An international initiative that seeks to promote the development and responsible use of AI, respecting human rights and diversity.
Website: https://gpai.ai - Research methodology on AI and inclusion (Digital Rights):
Developed by the organization Digital Rights to examine the use of AI technologies in Latin America, under human rights criteria.
Report: https://www.derechosdigitales.org/wp-content/uploads/ia-inclusion-metodologia.pdf - Case studies in Latin America:
- Brazil: Using AI to profile unemployed people and allocate benefits, raising concerns about discrimination and transparency.
Fountain: “Discriminatory algorithms in Brazil” – Digital Rights
https://www.derechosdigitales.org/12758/algoritmos-discriminatorios-en-brasil
- Brazil: Using AI to profile unemployed people and allocate benefits, raising concerns about discrimination and transparency.
Appendix 4: Practical examples of problems associated with AIs and search engines
Spread of Misinformation
One significant issue arises when AI systems mistakenly confuse one individual with another due to similar names. For instance, an AI might attribute criminal allegations to a person simply because their name closely resembles that of someone involved in a legal case. This type of error can cause unnecessary reputational harm, create confusion, and mislead others about the facts.
Another example involves AI systems offering outdated information. Many current generative AIs operate with data that is delayed by 30 days or more, which is particularly problematic when addressing rapidly evolving topics like economic policy or health guidelines. This lag can lead users to make decisions based on information that is no longer accurate or relevant.
Finally, a lack of geographic diversity in data sources compounds the problem. For instance, an AI might present an incomplete view of a global issue, such as climate change, by only referencing studies or perspectives from one region. This failure to include sources from multiple countries creates a biased narrative that overlooks critical variations in context and impact.
Algorithmic Biases
Biases within algorithms often arise from the limited or unbalanced datasets used to train them. For example, a search engine might disproportionately display articles from dominant Western media outlets when queried about international news. This lack of representation excludes perspectives from non-Western countries, limiting users’ ability to understand the broader global context.
Similarly, when searching for professions, an AI might prioritize stereotypical images, such as showing primarily male doctors or female nurses. These biased results reinforce harmful stereotypes and fail to reflect the diversity present in modern workplaces.
Unsubstantiated AI-Generated Opinions
Unverified AI-generated opinions are another major concern. For instance, an AI might suggest that a public official mishandled a crisis, relying on speculative or unverified sources rather than objective facts. Such baseless claims can distort public opinion, influence media narratives, and unfairly damage the reputation of the individual involved.
Outdated Content
Outdated information is an issue that consistently undermines the reliability of AI systems. For example, an AI providing financial advice might refer to stock market conditions or interest rates from a month ago, failing to reflect real-time market shifts. Users relying on this information could make poor investment decisions or miss critical opportunities.
Similarly, when dealing with legal or policy queries, an AI might cite legislation that has already been repealed or updated. For instance, a user seeking advice on immigration requirements could receive guidance based on outdated laws, potentially leading to procedural errors or delays.
Appendix 5: Resources to expand digital rights research
International organizations and projects:
- Electronic Frontier Foundation (EFF):
Defends digital privacy, freedom of expression and human rights in the digital environment.
Website: https://www.eff.org - Digital Rights:
Latin American organization dedicated to the promotion and defense of human rights in the digital environment.
Website: https://www.derechosdigitales.org - AI Now Institute:
Organization dedicated to studying the social implications of AI.
Website: https://ainowinstitute.org
Educational tools and courses:
- Course on digital literacy (European Digital Rights – EDRi):
Offers educational resources to understand digital rights.
Website: https://edri.org - Mozilla Foundation:
Promotes transparency and ethics in AI and digital technologies.
Website: https://foundation.mozilla.org
Relevant reports and studies:
- UNESCO: Recommendation on the Ethics of Artificial Intelligence (2021):
Provides a global framework for AI ethics.
Access: https://unesdoc.unesco.org/ark:/48223/pf0000377897 - Amnesty International:
Reports on the impact of AI on human rights, such as biases in facial recognition systems.
Access: https://www.amnesty.org/es/latest/research/
Final thoughts
The intersection between digital rights and artificial intelligence is a constantly evolving field that requires attention and coordinated action. As technologies advance, it is essential to ensure that their development and application respect and promote human rights.
Key areas to move forward:
- Digital inclusion: Ensure that all communities have equitable access to AI technologies, minimizing digital divides and avoiding exclusion.
- Algorithmic bias prevention: Implement practices to identify, mitigate and eliminate biases in AI models, ensuring fair and representative results.
- Transparency and algorithmic traceability: Promote openness in the processes and decisions of AI systems, allowing audits and facilitating understanding by users and regulators.
Multi-sector collaboration:
Cooperation between governments, international organizations, the private sector, academia and civil society is essential to develop regulatory and ethical frameworks that guide the responsible use of AI.
@ 26/11/2024 by Héctor Castillo “LinkedIn” – Noysi & AidaFramework.com Founder
Final Reflection
Ethical humans, when faced with uncertainty, often respond with humility: “I don’t know; let me check, and I’ll get back to you shortly.” This simple acknowledgment of limitations not only preserves trust but also ensures that the information provided is accurate and responsible.
If AIs were designed to follow this same principle instead of perpetuating “consented hallucinations,” the internet would avoid many of its most pressing risks. The refusal to admit uncertainty is paving the way for a digital ecosystem increasingly vulnerable to misinformation and mistrust. In fact, the solution could be as straightforward as teaching AIs to respond, “I don’t know; let me verify and provide you with accurate information later.”
So why isn’t this being implemented already? The answer lies in the race for immediacy, convenience, and perceived authority over accuracy. This choice prioritizes appearing capable over being correct, a trade-off that has profound consequences for the integrity of our information networks.
If we are to safeguard the future of the internet, the time to demand transparency and accountability from AI systems is now. A simple shift in design philosophy—embracing the ability to say “I don’t know”—could solve much of the chaos we are beginning to witness.
Comments
Este es un trabajo que merece una primer lectura general, pars luego comenzar una lectura reflexiva y comprensiva, para aprehenderlo e integrarlo en nuestros conectores de negocio y éticos. Gran aporte, muchas gracias!!!